Data Strategy for Early-Stage Startups
with Jacob Gustafsson (former Analytics Lead at Zettle by PayPal)
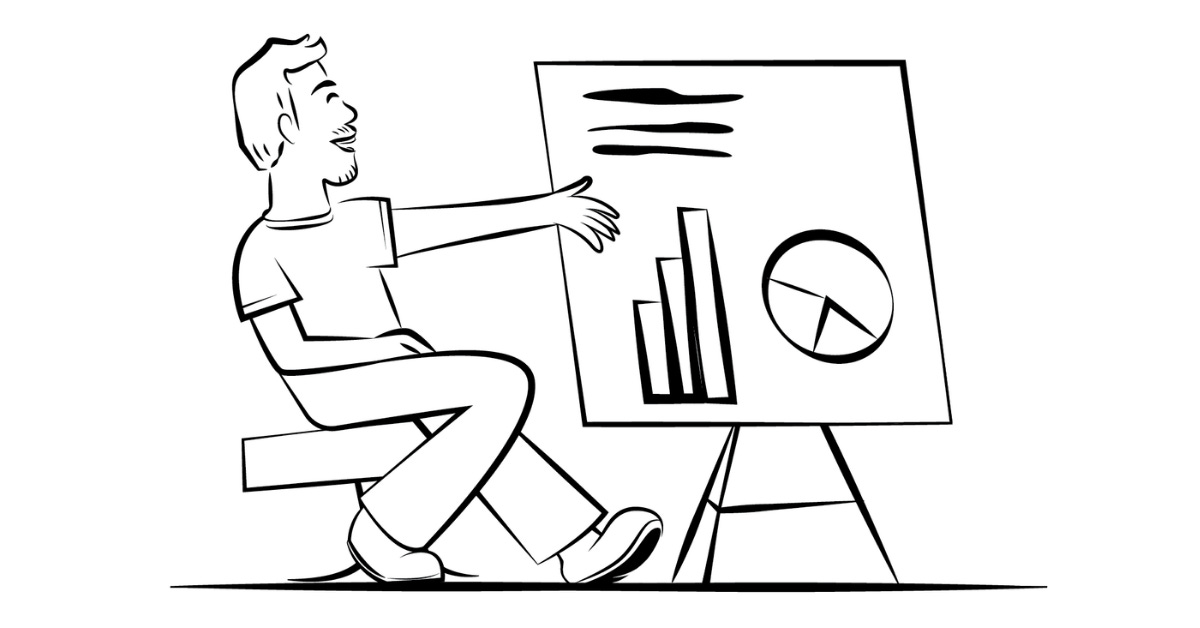
While the benefits of a solid data strategy are universal (better insights lead to better products, which leads to more growth opportunities for your business), it’s crucial to understand how the execution will vary depending on the stage of your company.
One of the main challenges for early-stage startups is that there is so much to do and so little time, money, and expertise. Yet, if data provides a valuable framework for thinking through priorities efficiently, it’s not immune from this same constraint. Hence, data strategy is usually an undertaking reserved for “after we've launched.”
To give some pointers, I asked a few questions to my former colleague Jacob Gustafsson, who built and led the analytics team at iZettle (now Zettle by PayPal).
On his journey
ED: According to this LinkedIn report, data-related jobs are some of the most in-demand right now. What led you to choose this career path "before it was cool"?
JG: I've always felt problem-solving and analysis to be stimulating. To figure things out through reasoning and logic has been fun for as long as I can remember.
However, joining the Growth Analytics team at a startup was more of a coincidence, I would say. I did not know of iZettle before joining, it appeared on a list of fast-growing startups and I saw an interesting job ad. Looking back, I did not completely know what I was getting into. But, it became clear pretty early that it was a good fit for me; I was very fortunate to be part of the journey at iZettle and learned a lot.
Data analytics will only increase in importance for tech companies going forward; it will be interesting to see how the space develops. I'm happy to be part of it.
On data, or lack thereof
ED: In your profile introduction, you write "helping startups become data-led and grow through user insights.” Can you expand on that? What are the main problems startups have when it comes to data and analytics?
JG: Startups have many challenges. On the one hand, they need to iterate on the product to find a good fit in the market. They also need to evaluate the distribution channels and find a sustainable way of acquiring new customers. On the other hand, since they are at an early stage, they don't yet have much data at hand. So it's harder to make data-led decisions. This is a crucial problem to solve; you need to be creative in the ways you gather data and systematic in the way you use it.
Early on, you sometimes need to "hack" your way to get the insights you need before getting more structured about data pipelines, instrumentation, and BI-tooling. I help companies with tools and techniques that they can use to get those insights and ultimately grow their product. Some hacks can be:
Generate insights through micro surveys within your product, or actively reach out to customers and do interviews that yield valuable qualitative insight. The Superhuman case is a famous example of reaching P/M fit through insights early on). So the point here is, if you don’t get the data through product usage events, ask your customers smart questions.
Use the scientific method without doing controlled experiments. Work with hypotheses, and think about observations that can reject your hypotheses, and iterate. A low volume of data is no excuse for being unscientific about how you build knowledge.
Store or save the learnings and make it accessible across your team, that knowledge base will compound in value over time.
On strategy and structure
ED: Technical debt is a common challenge in software development. The same concept applies to analytics. How can startups avoid "data debt"?
JG: Early on, you should do two things:
1. Get insights using data that you have to drive decisions.
2. Think strategically about how your data stack should look like given the applications you foresee 3-5 years ahead, and build it in parallel.
Data debt can be mitigated by (2) above. I believe designing a robust pipeline, data warehouse, transformations, and BI-tooling is necessary to get to a point where data-led decision-making can really scale in the organization.
In addition to the data stack, tracking and instrumentation are key. I've learned to never underestimate the importance of structured naming conventions and KPI definitions. It will save a lot of time and money to do this consistently from day 1. It does not have to be complex at all, but there needs to be a way of naming events and one source of truth for the main KPIs; you will have to do it sooner or later.
One example from previous experience was that, during a project to generate insight about how a particular metric developed over time, while the analysis was done and results shared, the stakeholders involved realized that they had two different ideas about what the KPI itself meant. Leading to confusion and more work. I’ve also seen cases where KPIs started to emerge organically in different teams that contradicted each other in their definition, leading to that any meeting where these KPIs were discussed had to start with a lengthy “how do you define that” discussion. It’s a good sign that teams want to define meaningful KPIs, but a central way of defining, aligning, and storing these definitions is needed.
On the abundance of tools
ED: As famously illustrated here, the MarTech landscape keeps on growing, and choosing the right analytics tools can quickly become a rabbit hole for startup teams. What stack do you generally recommend for early-stage startups?
JG: The number of tools is increasing, and the quality of those tools as well.
The most important thing to consider when choosing tools is to make sure you understand what problems you are solving. Pipelining, Warehousing, Transforming, Visualizing are examples of problems, and there are various providers within each of those with specific nuances that could be a good fit.
Within the transformation step, I think getdbt from Fishtown Analytics is an exciting concept. It makes data analysts and data scientists work more like developers in that they use code and version control to do the transformations and build models. With this, analysts get more influence and autonomy on the data structure. I think this trend will accelerate, and companies, where analysts build tools in addition to consuming and analyzing data, will be successful.
Some of the most used tools, ordered by category, are:
Pipelining: Fivetran, Segment
Data warehousing: Snowflake, Google BigQuery, AWS Redshift
Transforming: getdbt (as mentioned above)
Visualisation: Looker, Tableau, Amplitude
On building a data-led culture
ED: Most startups don't have the luxury to have a data analyst early on. So when do you think is a good time to hire your first data analyst and build a team?
JG: In the beginning, everyone is an analyst.
Companies should use insights for decision-making from day 1. But you don't need to have "Analyst" in your title to do that. The first PM of a company probably needs to take on an analyst’s hat at times to evaluate a launch, prepare for new markets, etc. The first dedicated analyst probably comes when it's clear that the data has the potential to drive significant added value through insights. And this person should probably be more of a generalist who can support both commercial and product teams and be a "full-stack analyst" with some engineering skills. I've seen companies wait until Series C for hiring an analyst, and some are doing it at the Seed stage; it depends on the nature of the business. The one clear thing, though, is that every company should make an active decision on whether to prioritize the analytics/insights investments or not at any given stage.
On career advice
ED: What tips would you give to future data analysts?
JG: Learn broadly in the beginning – some data engineering, some analysis/scripting, some dashboarding, and communication. Getting a feel for all of these areas will make it easier to decide what to specialize in (unless you want to stay full-stack). Don't forget the communication part; a good analyst can have much more impact if insights are communicated in a clear way that decision-makers understand. I've seen cases where communication did not work or was not focused on, and the result was simply that those insights were not used.
Secondly, prepare to work on culture as well. Stakeholders within a company need to be educated around how to be data-led, and the culture is a deciding factor if companies will leverage analytics or not.
You are an analyst but also a driver of the data-led culture.
I hope this post was helpful and valuable. If you have any questions or would like to talk data and analytics with Jacob, you can connect with him on LinkedIn.
Thank you for reading! Wishing you all a fantastic summer ⛱